In-store analytics is the process of collecting, analyzing, and using data about customer behavior and interactions in physical retail spaces in order to improve business performance and drive results. The role of in-store analytics in the age of e-commerce is more important than ever, as it allows retailers to understand and optimize the in-store customer experience in a way that can help to drive better results.
The importance of in-store analytics in the age of e-commerce cannot be overstated. With the rise of online shopping and the increasing competition from e-commerce companies, it is more important than ever for brick-and-mortar retailers to understand and optimize the in-store customer experience in order to drive results. By collecting and analyzing data about customer behavior and interactions in physical retail spaces, businesses can gain insights into what works and what doesn’t and can use this information to make informed decisions about how to improve the in-store customer experience.
This article will explore the importance of in-store analytics in the age of e-commerce, and will discuss the various types of in-store analytics that retailers can use. We will also cover techniques for collecting and analyzing in-store data, as well as best practices for using in-store analytics to drive revenue and sales.
The importance of in-store analytics in the age of e-commerce
In the age of e-commerce, in-store analytics is becoming more important as retailers look for ways to stay competitive and attract customers in a digital landscape.
One of the main ways in-store analytics can drive results is by helping retailers better understand and optimize their in-store customer experience. By analyzing data on customer foot traffic, browsing and purchase patterns, and other metrics, retailers can identify areas for improvement and make informed decisions about store layout, product placement, and other factors that can impact the customer experience.
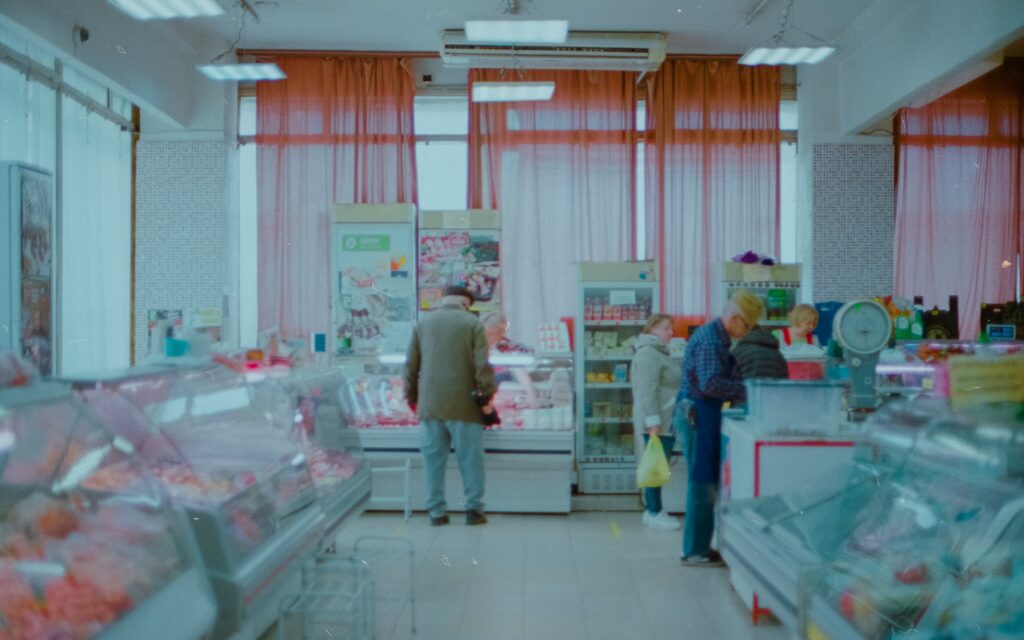
In-store data can also help retailers optimize their inventory management and supply chain operations. By analyzing data on sales, returns, and other key metrics, retailers can better predict demand and adjust their inventory levels accordingly, leading to reduced waste and cost savings.
Examples of successful in-store analytics in the age of e-commerce include retailers using data to personalize the in-store experience for customers, such as by using customer data to recommend products or personalized discounts. Other retailers have used in-store data to optimize their store layouts and product placements to drive sales, and to improve their supply chain operations by analyzing data on sales, returns, and other key metrics.
Overall, in-store analytics plays a critical role in helping retailers stay competitive and drive results in the age of e-commerce. By collecting and analyzing data from their physical retail locations, retailers can gain valuable insights that can help them optimize their operations and improve the customer experience.
Types of in-store analytics
In-store data is becoming an increasingly important tool for retailers looking to optimize their operations and drive results.
There are several types of in-store analytics that retailers can use to gain insights into their business and make informed decisions.
Customer traffic and footfall analytics
One type of in-store analytics is customer traffic and footfall analytics. This involves collecting and analyzing data on the number of customers visiting a store, as well as their movements and behaviors within the store. This data can help retailers understand which areas of the store are most popular, identify trends in customer behavior, and optimize the store layout and product placements to drive sales.
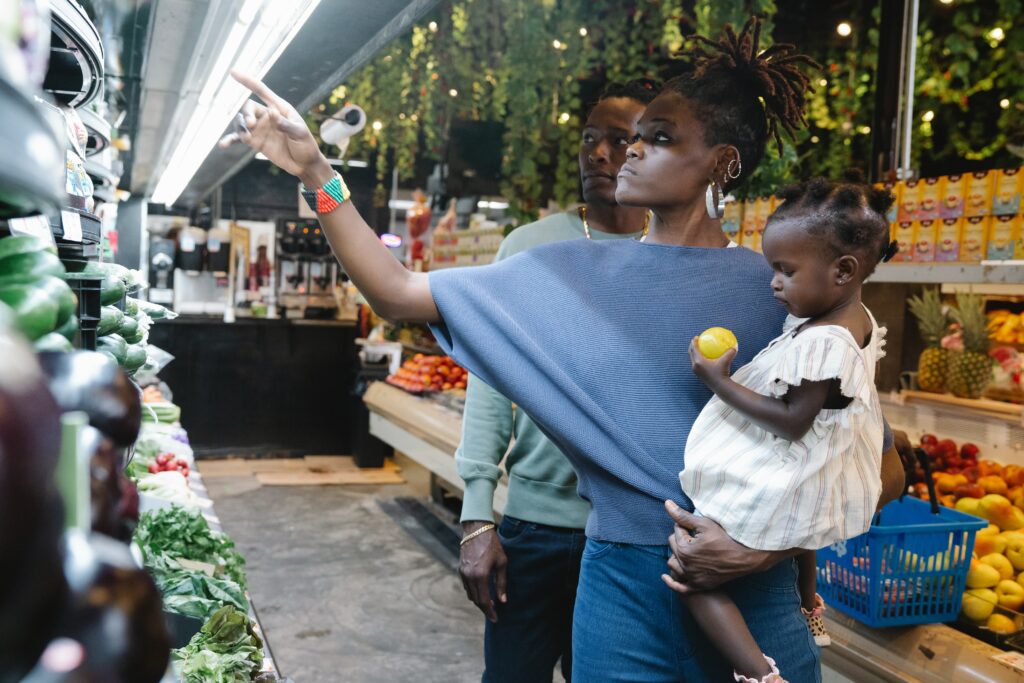
Customer behavior analytics
Customer behavior data is another important type of in-store analytics. This involves collecting and analyzing data on how customers interact with products, browse the store, and make purchases. This data can help retailers understand what drives customer behavior and make informed decisions about the types of products and marketing efforts that are most effective at driving sales.
Sales and revenue analytics
Sales and revenue data is another key type of in-store analytics. This involves collecting and analyzing data on sales and revenue, as well as key metrics such as average transaction size and customer lifetime value. This data can help retailers understand what drives sales and identify opportunities for growth.
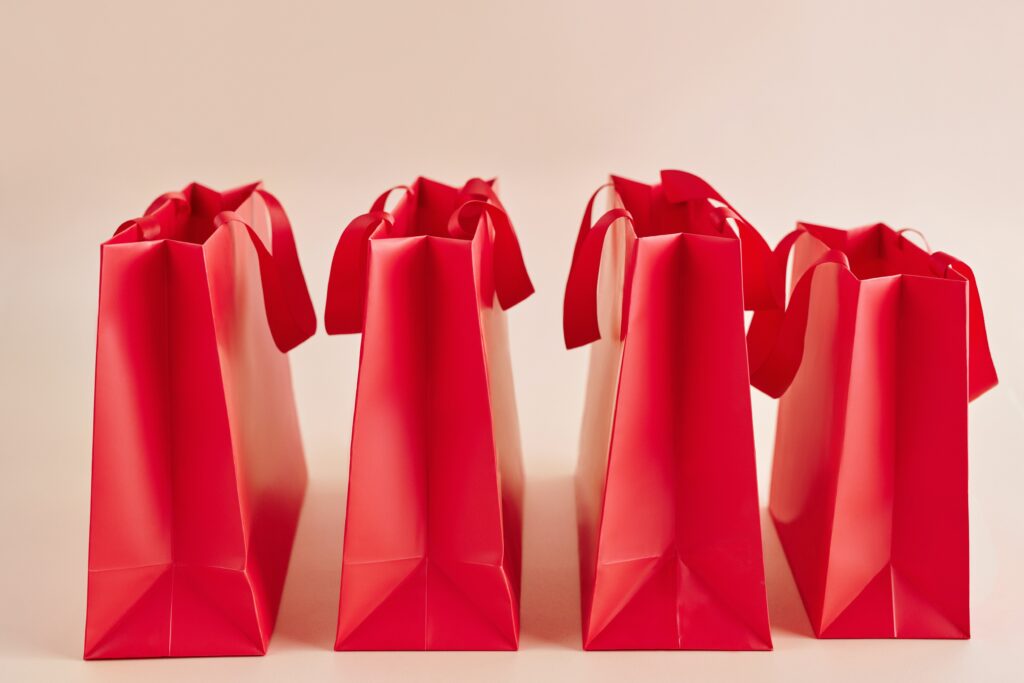
In-store Marketing Analytics
In-store marketing analytics is another important type of in-store analytics. This involves collecting and analyzing data on the effectiveness of in-store marketing efforts. For example, signage, promotional materials, and in-store events. This data can help retailers understand what marketing efforts are effective at driving sales. Ultimately, make informed decisions about future marketing efforts.
By collecting and analyzing data from their physical retail locations, retailers can gain valuable insights into revenue trends. Additionally, learn the effectiveness of marketing efforts, enabling them to make informed decisions and drive success.
Techniques for collecting and analyzing in-store analytics
There are several techniques that retailers can use to collect and analyze in-store analytics. These techniques can help retailers gain valuable insights into the effectiveness of marketing efforts, enabling them to optimize their operations and drive results.
Use of in-store tracking technology
One technique for collecting and analyzing in-store data is the use of in-store tracking technology. This can include sensors, beacons, and other types of technology that collect data on customer foot traffic, behavior, and purchases. This data can be analyzed to understand customer behavior and identify trends and patterns that can inform business decisions.
Customer Data analysis
Customer data analysis is another technique for collecting and analyzing in-store data. This involves collecting and analyzing data on customer demographics, preferences, and behaviors, as well as data on sales and revenue. This data can help retailers understand their customer base and identify opportunities for growth.
Market research
Market research is another relevant technique for collecting in-store analytics. This can include surveys, focus groups, and other types of research to gather insights into customer attitudes and behaviors. This data can be combined with data from in-store tracking technology and customer data analysis. The result is to gain a more complete understanding of customer behavior and business decisions. For more information about Market Research, read this complete guide for Market Research for Retail Businesses
By combining data from in-store tracking technology, customer data analysis, and market research, retailers gain valuable insights into customer behavior. As mentioned before, they can also learn the effectiveness of marketing efforts, optimize their operations, and drive results.
Best practices for using in-store analytics
Using in-store analytics effectively requires following best practices. Additionally, ensuring that the data being collected and analyzed is relevant, accurate, and actionable. Some best practices include:
- Identifying and prioritizing key metrics. It’s important to identify the most important metrics to track and prioritize when collecting and analyzing data. This can help retailers focus on the data that is most relevant and valuable to their business.
- Integrating in-store analytics with online analytics. In the age of e-commerce, it’s important to consider both online and in-store data when making business decisions. By integrating in-store data with online data, retailers can gain a more complete understanding of customer behavior. Consequently, they can optimize their operations across all channels.
- Testing and optimizing in-store data strategies. It’s important to regularly test and optimize strategies to ensure that they are effective. This can involve conducting experiments to test different approaches and using data to inform decision-making.
Following the above best practices can help retailers make informed decisions that drive results and optimize their operations. Identify and prioritize key metrics, integrate in-store data with online data, and test and optimize strategies.
Conclusion
In conclusion, in-store analytics plays a critical role in helping retailers stay competitive and drive results. By collecting and analyzing data from their physical retail locations, retailers can gain valuable insights. Customer behavior, sales and revenue trends, and the effectiveness of marketing efforts. This data can inform business decisions and help retailers optimize their operations and improve the customer experience.
As e-commerce continues to grow and evolve, retailers must consider the role of in-store data in their overall strategy. This involves leveraging new technologies for collecting and analyzing data, as well as testing and optimizing strategies to ensure that they are effective.
Shoppermotion’s solutions are designed to help retailers measure the performance of their offline campaigns by collecting in-store data. By using Shoppermotion‘s solutions, retailers can gain insights into customer behavior and ultimately, optimize their operations and drive results.